Author: Brigitte Durieux
Posted: October 2019
Context
Recent qualitative studies have implicated uncertainty as a barrier within Palliative Care (PC) (McIlvennan, 2016; Basu, 2018; Chow, 2018; Kim, 2018). In the serious illness context, there exists inherent prognostic uncertainty (Mishel, 1981; McCormick, 2002; Oishi, 2014) which must be discussed in conversations between patient and clinician (e.g. high-risk/high-payoff treatment trials, prognosis, possibility for procedure complications, next-step options). Recent literature has also described the presence of both verbally-expressed and thematic uncertainty acting influentially on patient experience and decision-making (Brashers, 2001; Etkind, 2016; Basu, 2018). It is well known that the framing of information can affect its reception; this remains the case with expressions of uncertainty, as different framing styles can be used to imply information that would otherwise be stated outright. Uncertainty is significant and prevalent in PC for patients with advanced cancer (Temel, 2016), and managing that uncertainty and its transmission appears to be one mechanism underlying PC impact on improving quality of life (Gramling, 2018).
Uncertainty Management is a central conceptual feature of patient-centered communication in cancer care (Epstein, 2007), but little is empirically known about uncertainty management (Epstein, 2013; Gramling, 2013). Understanding this complex idea will require automation or semi-automation of conversation feature measurement in large sample studies (Tulsky, 2017).
As is the case with any variables, language measures must be backed by a conceptual framework (i.e., one must be able to recognize or mark something to quantify it). As a host of words may all signify uncertainty, all possible target uncertainty terms can be combined to a corpus. This corpus can be used by Natural Language Processing (NLP) to generate a sum frequency measure for all uncertainty words within the conversation. We have created a corpus of uncertainty language with conceptual basis to provide us with measurable variables (frequency, proportion within the conversation, etc.). The resulting data allow us to compare a conversation’s uncertainty scores with other conversational features and/or survey data.
Methods
Word List Generation: The goal was to draft a corpus for conversational uncertainty within the Palliative Care context. We created an initial list of 51 Uncertainty Words from relevant literature (Vincze, 2014; Strekalova, 2017) to detect uncertainty words in medical contexts, and expanded this list to 186 alternate forms (plurals, etc.). Next, we identified synonyms for each term using WordNet (Princeton, 2010), a lexical database for the English language, and the Random House Digital Thesaurus. From this list of 1,229 terms, we removed any words that did not appear at least once in the PCCRI textual database, and removed any words that were not synonymous with the target definition of the associated original term. We collapsed variants of the same word into their stems (to avoid unintentionally classifying variants of the same word into different uncertainty sub-types) to get a dictionary of 246 terms. The whole lab group met to adjudicate the relevance of each term within the dictionary, resulting in a list of 155 most relevant terms. Terms that did not require adjudication, as there was initial group consensus on relevance, encompassed a list of 113 words, which we refer to as the Strong Subgroup.
Word Finder: First, we identified all words considered speech (i.e., we excluded transcript labels for non-speech events, such as coughing or laughing). Second, we stripped all leading and trailing whitespaces and punctuation surrounding each word to create a simple ordered string of words separated by one space. Third, we used regular expressions and our uncertainty search terms to identify all instances of the target uncertainty language in the full PCCRI text dataset (by conversation and by speaker (i.e. patient/family or clinician)) within each conversation. We wrote into the script a list of exception rules to keep irrelevant words with a target stem from being added, and to avoid negations (target words with altered meaning due to a preceding ‘no’, ‘un’, ‘not’, or ‘non’). We manually checked five full transcripts using the Uncertainty Word List and identified no errors in the algorithm.
Defining Uncertainty Subtypes: Determining subgroups of uncertainty was an iterative process. We first explored the existing groupings presented in existing literature. Notably, we began working off the groups defined in a 2017 Strekalova et al. paper: possibility-indicating verbs, hedging verbs, qualifiers, direct expressions of uncertainty, and question-asking. We also explored groupings based on Han’s Taxonomy of Uncertainty (Han, 2011). In Han’s conceptual model, uncertainty is organized into three primary domains: ambiguity, complexity and probability. Ambiguity involves information subject to interpretation (whether that is due to imprecision, conflicting data/opinions, or a lack of information). Complexity is implicated in situations involving an array of determining factors and/or interpretive signals. Probability concerns the likelihood of a potential future event to occur (i.e. indeterminacy of outcome) (Babrow, 1998; Han, 2011). To look for natural clusters of uncertainty terms based on our terms’ definitions, we also used a visualization program, Gephi (Bastian, 2009), to graph the “proximity” of one term to another using strength of equivalence from synonym directories. Ultimately, we settled on uncertainty subtypes of Hedging Terms, Possibility Indicators, Confusion Terms, and Prognosis Uncertainty Terms.
Subtype Definitions
Hedging Terms: words used to suggest something rather than state it; by using ‘think’ instead of ‘know’, one adds some level of uncertainty to the statement that follows. Hedging implies speculation (Vincze, 2014).
Possibility Indicators: words giving some uncertain probability or possibility to the associated statement. These include modal verbs.
Confusion Terms: verbal expressions of direct confusion, complexity, a lack of knowledge, or uncertainty.
Uncertainty Corpora
Team-Adjudicated Uncertainty Corpus: “Strong Subgroup” (*= stem)
a bit | contemplate | hope | most likely | presume | take a chance |
a little | convoluted | hypothesi* | most of the time | presuppose | theor* |
allegedly | could | imagine | nearly | probability | think |
allude to | curiosity | imply | not certain | probabl* | try |
ambiguity | deem | in all likelihood | not convinced | prognosticate | uncertain |
anticipate | discombobulate | in all probability | not know | quite | unclear |
approximately | do not understand | incertain | not sure | reasonable | unconvinced |
assess | don’t know | inconstant | often | relatively | undecided |
baffle | don’t understand | infer | perceive | risk | unexpected |
befuddle | doubt | kind of | perchance | roughly | unlikely |
bewilder | dubious | likel* | perhaps | seem | unpredictable |
call into question | dumbfound | may | perplex | should | unsure |
chance | estimate | maybe | plausibl* | slightly | usually |
changeable | expect | might | ponder | somewhat | vary |
complex | feasibl* | misinterpret | possibility | sort of | virtually |
complicated | foresee | misjudge | possibl* | speculate | whether |
confound | generally | mistrust | postulate | suggest | whether or not |
confus* | guess | misunderstand | potential | suppose | worr* |
consider | hint | mixed up | predict | suspect |
Uncertainty Subtype Corpus: “Hedging Terms” (*= stem)
allude to | doubt | hope | misjudge | presuppose | suppose |
anticipate | estimate | hypothesi* | perceive | prognosticate | suspect |
assess | expect | imagine | ponder | risk | theor* |
consider | foresee | imply | postulate | seem | think |
contemplate | guess | infer | predict | speculate | worr* |
deem | hint | misinterpret | presume | suggest |
Uncertainty Subtype Corpus: “Possibility Indicators” (*= stem)
could | feasibl* | may | nearly | possibility | probabl* |
chance | in all likelihood | maybe | perchance | possibl* | reasonable |
changeable | in all probability | might | perhaps | potential | |
generally | likel* | most likely | plausibl* | probability |
Uncertainty Subtype Corpus: “Confusion Terms” (*= stem)
ambiguity | confound | dubious | mixed up | perplex | unlikely |
baffled | confus* | dumbfound | not certain | uncertain | unpredictable |
befuddle | convoluted | incertain | not convinced | unclear | unsure |
bewilder | discombobulate | inconstant | not know | unconvinced | |
complex | don’t know | mistrust | not sure | undecided | |
complicated | don’t understand | misunderstand | not understand | unexpected |
Initial Results
Figure 1: Uncertainty distribution becomes tighter as search term list is revised
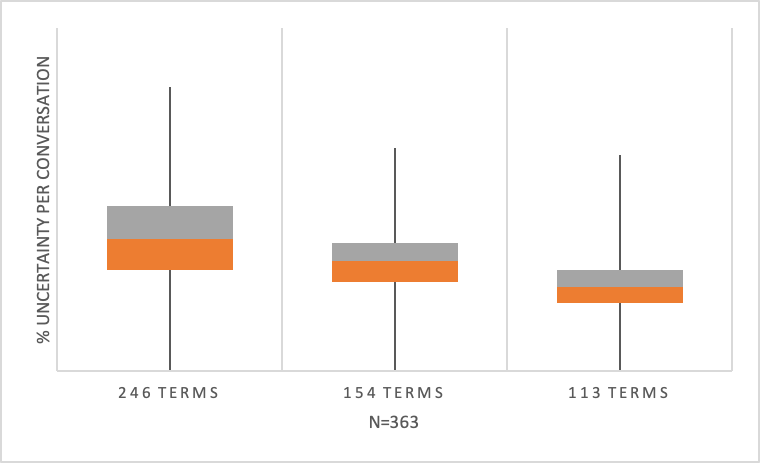
Figure 2: Average percent uncertainty (percent uncertainty words used out of all words in each conversation per subgroup) per conversation, across 231 palliative care consults
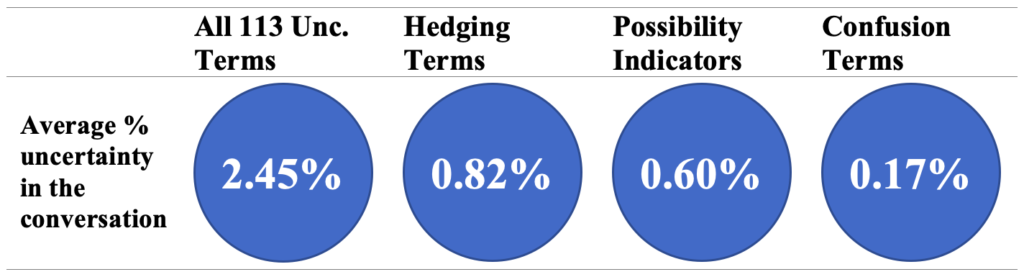
References
Babrow AS, Kasch CR, Ford LA. The many meaning of uncertainty in illness: toward a systematic accounting. Health Commun. 1998;10(1):1–23.
Brashers DE. Communication and uncertainty management. J Commun 2001; 51: 477–497.
Bastian M., Heymann S., Jacomy M. (2009). Gephi: an open source software for exploring and manipulating networks. International AAAI Conference on Weblogs and Social Media.
Basu S, Swil K. Paediatric advance care planning: Physician experience and education in initiating difficult discussions. J Paediatr Child Health. 2018 May;54(5):510-514.
Chow J, Senderovich H. It’s Time to Talk: Challenges in Providing Integrated Palliative Care in Advanced Congestive Heart Failure. A Narrative Review. Curr Cardiol Rev. 2018;14(2):128-137.
Epstein RM, Street RL. Patient-Centered Communication in Cancer Care. Bethesda, MD: National Institutes of Health; 2007.
Epstein RM, Gramling RE. What is shared in shared decision making? Complex decisions when the evidence is unclear. Med Care Res Rev. 2013;70(1 Suppl):94S-112S.
Etkind, S. N., Bristowe, K., Bailey, K., Selman, L. E., & Murtagh, F. E. (2016). How does uncertainty shape patient experience in advanced illness? A secondary analysis of qualitative data. Palliative medicine, 31(2), 171-180.
Gramling R, Carroll T, Epstein R. Prognostication in Advanced Illness. In: Goldstein N, Morrison RS, eds. Evidence-Based Practice of Palliative Medicine: Elsevier Press; 2013.
Gramling R, Stanek S, Han PKJ, et al. Distress Due to Prognostic Uncertainty in Palliative Care: Frequency, Distribution, and Outcomes among Hospitalized Patients with Advanced Cancer. J Palliat Med. 2018;21(3):315-321.
Han PK, Klein WM, Arora NK. Varieties of uncertainty in health care: a conceptual taxonomy. Med Decis Making. 2011;31(6):828-838.
Kim JW, Atkins C, Wilson AM. Barriers to specialist palliative care in interstitial lung disease: a systematic review. BMJ Support Palliat Care. 2018 Nov 21. pii: bmjspcare-2018-001575.
McCormick KM. A concept analysis of uncertainty in illness. J Nurs Scholarsh. 2002;34(2):127-31.
McIlvennan CK, Allen LA. Palliative care in patients with heart failure. BMJ. 2016 Apr 14; 353: i1010.
Mishel MH. The Measurement of Uncertainty in Illness. Nurs Res 1981; 30(5): 258–263.
Oishi A, Murtagh FE. The challenges of uncertainty and interprofessional collaboration in palliative care for non-cancer patients in the community: a systematic review of views from patients, carers and health-care professionals. Palliat Med 2014; 28: 1081–1098.
Princeton University “About WordNet”. WordNet, version 3.1. Princeton University. 2010.
(2018). Random House Webster’s Unabridged Dictionary. New York, Random House Reference.
Strekalova YA, James VS. Language of Uncertainty: the Expression of Decisional Conflict Related to Skin Cancer Prevention Recommendations. J Cancer Educ. 2017;32(3):532-536.
Temel JS, Shaw AT, Greer JA. Challenge of Prognostic Uncertainty in the Modern Era of Cancer Therapeutics. J Clin Oncol. 2016.
Thesaurus.com. 2018. https://www.thesaurus.com (28 Nov 2018).
Tulsky JA, Beach MC, Butow PN, et al. A Research Agenda for Communication Between Health Care Professionals and Patients Living With Serious Illness. JAMA Intern Med. 2017.
Vincze V. Uncertainty Detection in Natural Language Texts. Szeged, Hungary: Research Group on Artificial Intelligence, University of Szeged; 2014.